Improving Generalization in Game Agents with Data Augmentation in Imitation Learning
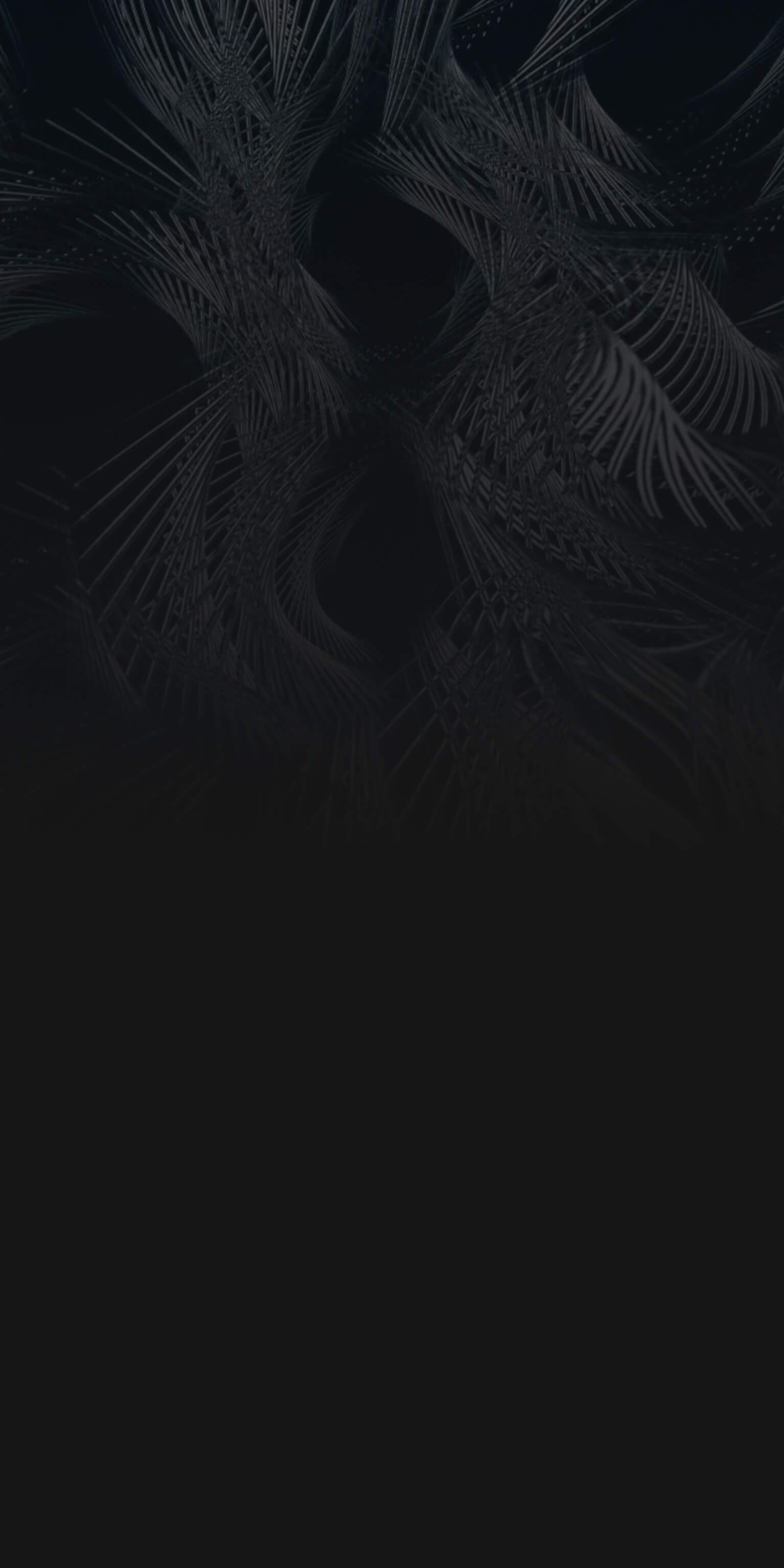
This presentation was delivered at the IEEE World Congress on Computational Intelligence, which was held on 5 July 2024 in Yokohama, Japan.
Authors: Derek Yadgaroff, Alessandro Sestini, Konrad Tollmar, Ayça Özçelikkale, and Linus Gisslén. Presented by Alessandro Sestini.
How do we efficiently train in-game AI agents to handle new situations that they haven’t been trained on?
Imitation learning is an effective approach for training game-playing agents and, consequently, for efficient game production. However, generalization – the ability to perform well in related but unseen scenarios – is an essential requirement that remains an unsolved challenge for game AI. Generalization is difficult for imitation learning agents because it requires the algorithm to take meaningful actions outside of the training distribution.
In this paper, we propose a solution to this challenge. Inspired by the success of data augmentation in supervised learning, we augment the training data so the distribution of states and actions in the dataset better represents the real state-action distribution.
This study evaluates methods for combining and applying data augmentations to improve the generalization of imitation learning agents. It also provides a performance benchmark of these augmentations across several 3D environments. These results demonstrate that data augmentation is a promising framework for improving generalization in imitation learning agents.
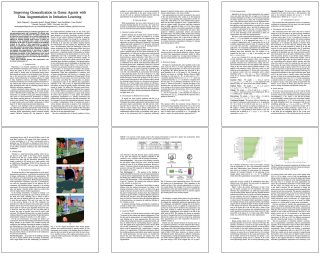
