EGSR 2022: Scalar Spatiotemporal Blue Noise Masks
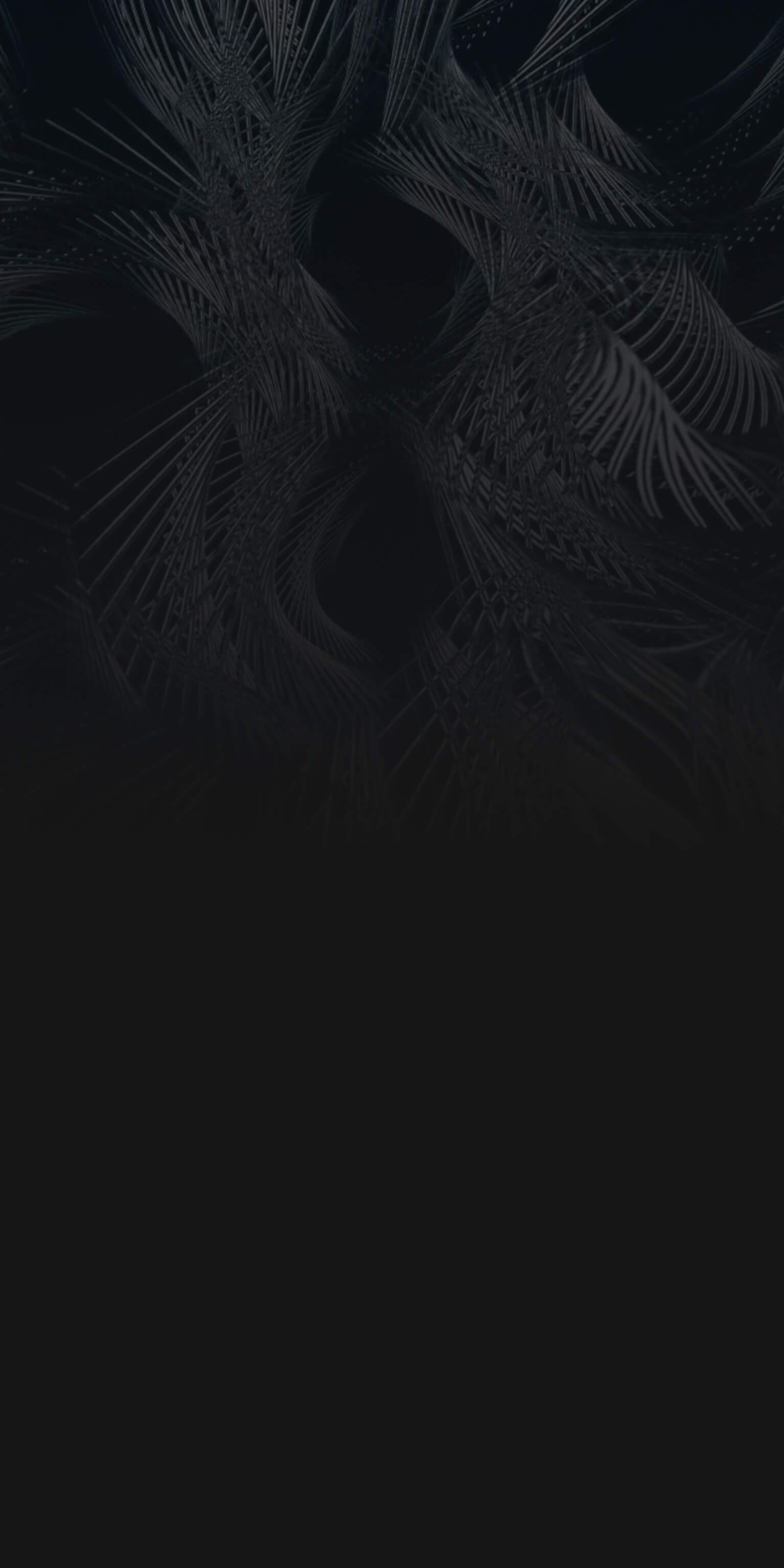
This is a research presentation from EGSR 2022 about using blue noise textures as a dithering technique to reduce noise in 3D rendering.
Download the presentation slides
Abstract: Blue noise error patterns are well suited to human perception, and when applied to stochastic rendering techniques, blue noise masks (blue noise textures) minimize unwanted low-frequency noise in the final image.
Current methods of applying blue noise masks at each frame independently produce white noise frequency spectra temporally. This white noise results in slower integration convergence over time and unstable results when filtered temporally. Unfortunately, achieving temporally stable blue noise distributions is non-trivial since 3D blue noise does not exhibit the desired 2D blue noise properties, and alternative approaches degrade the spatial blue noise qualities.
We propose novel blue noise patterns that, when animated, produce values at a pixel that are well distributed over time, converge rapidly for Monte Carlo integration, and are more stable under TAA, while still retaining spatial blue noise properties. To do so, we propose an extension to the well-known void and cluster algorithm that reformulates the underlying energy function to produce spatiotemporal blue noise masks. These masks exhibit blue noise frequency spectra in both the spatial and temporal domains, resulting in visually pleasing error patterns, rapid convergence speeds, and increased stability when filtered temporally.
We demonstrate these improvements in a variety of applications, including dithering, stochastic transparency, ambient occlusion, and volumetric rendering. By extending spatial blue noise to spatiotemporal blue noise, we overcome the convergence limitations of prior blue noise works, enabling new applications for blue noise distributions.
